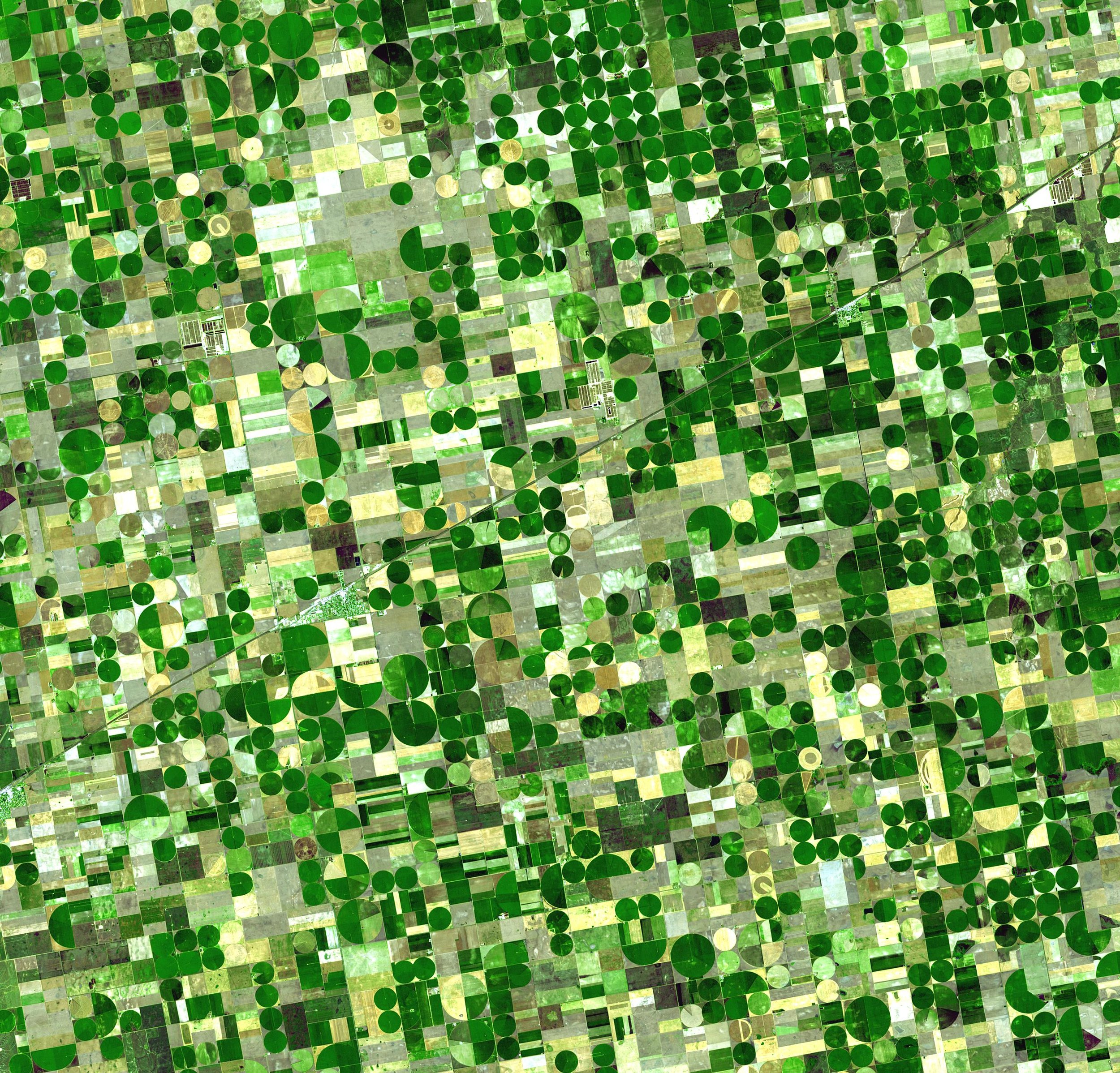
NASA Harvest Partner Research
(*Denotes Harvest Funding)
-
*Detection and mapping of artillery craters with very high spatial resolution satellite imagery and deep learning
Duncan, E.; Skakun, S.; Kariryaa, A.; Prishchepov, A. 2024. Detection and mapping of artillery craters with very high spatial resolution satellite imagery and deep learning. Science of Remote Sensing. doi.org/10.1016/j.srs.2023.100092
-
*Preseason maize and wheat yield forecasts for early warning of crop failure
Anderson, W.; Shukla, S.; Verdin, J.; Hoell, A.; Justice, C.; Barker, B.; Slinski, K.; Lenssen, N.; Lou, J.; Cook, B.; McNally, A. 2024. Preseason maize and wheat yield forecasts for early warning of crop failure. Nature Communications. doi.org/10.1038/s41467-024-51555-8
-
*Estimation of sunflower planted areas in Ukraine during full-scale Russian invasion: Insights from Sentinel-1 SAR data
Qadir, A.; Skakun, S.; Becker-Reshef, I.; Kussul, N.; Shelestov, A. 2024. Estimation of sunflower planted areas in Ukraine during full-scale Russian invasion: Insights from Sentinel-1 SAR data. Science of Remote Sensing. doi.org/10.1016/j.srs.2024.100139
-
*Further adoption of conservation tillage can increase maize yields in the western US Corn Belt
Cambron, T.; Deines, J.; Lopez, B.; Patel, R.; Liang, S.; Lobell, D. 2024. Further adoption of conservation tillage can increase maize yields in the western US Corn Belt. Environmental Research Letters. doi.org/10.1088/1748-9326/ad3f32
-
Digital innovations for monitoring sustainability in food systems
Meemken, EM., Becker-Reshef, I., Klerkx, L. et al. Digital innovations for monitoring sustainability in food systems. Nat Food 5, 656–660 (2024). https://doi.org/10.1038/s43016-024-01018-6
-
*Climatic stresses and rural emigration in Guatemala.
Britos, Braulio; Hernandez, Manuel A.; Puricelli, Estefania; and Sahajpal, Ritvik. 2023. Climatic stresses and rural emigration in Guatemala. Project Note. Washington, DC; College Park MD: International Food Policy Research Institute (IFPRI); NASA Harvest. https://doi.org/10.2499/p15738coll2.136920
-
Satellite forecasting of crop harvest can trigger a cross-hemispheric production response and improve global food security.
Tanaka, T., Sun, L., Becker-Reshef, I. et al. Satellite forecasting of crop harvest can trigger a cross-hemispheric production response and improve global food security. Commun Earth Environ 4, 334 (2023). https://doi.org/10.1038/s43247-023-00992-2
-
Matching satellite-based crop classification with sample-based area estimation.
Li, H; et al. Development of a 10-m resolution maize and soybean map over China: Matching satellite-based crop classification with sample-based area estimation. Remote Sensing of Environment. 2023. https://doi.org/10.1016/j.rse.2023.113623
-
Earth observations into action: the systemic integration of earth observation applications into national risk reduction decision structures.
Borges, D.E.; et al. Earth observations into action: the systemic integration of earth observation applications into national risk reduction decision structures. Disaster Prevention and Management. 2023.
-
L-Band ALOS2-PALSAR2 for Discontinuous Permafrost Mapping in Peatland Regions.
Touzi, R.; Pawley, S.M.; Wilson, P.; Jiao, X.; Hosseini, M.; Shimada, M. Polarimetric L-Band ALOS2-PALSAR2 for Discontinuous Permafrost Mapping in Peatland Regions. Remote Sensing. 2023; 15(9):2312. https://doi.org/10.3390/rs15092312https://doi.org/10.3390/rs15092312
-
*Field-scale dynamics of planting dates in the US Corn Belt from 2000 to 2020.
Deines, J., Swatantran, A., Ye, D., Myers, B., Archontoulis, S., Lobell, D. (2023). Field-scale dynamics of planting dates in the US Corn Belt from 2000 to 2020. Remote Sensing of Environment. https://doi.org/10.1016/j.rse.2023.113551
-
*Crop Type Maps for Operational Global Agricultural Monitoring. Scientific Data.
Becker-Reshef, I., Barker, B., Whitcraft, A. et al. Crop Type Maps for Operational Global Agricultural Monitoring. Scientific Data. 10 (172). https://doi.org/10.1038/s41597-023-02047-9
-
Considerations for AI-EO for agriculture in Sub-Saharan Africa. Environmental Research Letters.
Catherine Nakalembe and Hannah Kerner. (2023). Considerations for AI-EO for agriculture in Sub-Saharan Africa. Environmental Research Letters. 18 (4). https://doi.org/10.1088/1748-9326/acc476
-
The Rise and Volatility of Russian Winter Wheat Production.
*Christian A., Skakun, S., Becker-Reshef, I. (2022). The Rise and Volatility of Russian Winter Wheat Production. Environ. Research Communications. 4 (10). https://doi.org/10.1088/2515-7620/ac97d2
-
Recent cover crop adoption is associated with small maize and soybean yield losses in the United States.
*Deines, J., Guan, K., Lopez, B., Zhou, Q., White, C., Wang, S., Lobell, D. (2023). Recent cover crop adoption is associated with small maize and soybean yield losses in the United States. Global Change Biology, 29 (3). https://doi.org/10.1111/gcb.16489
-
Near- to long-term measures to stabilize global wheat supplies and food security.
Bentley, A.R., Donovan, J., Sonder, K. et al. Near- to long-term measures to stabilize global wheat supplies and food security. Nat Food 3, 483–486 (2022). https://doi.org/10.1038/s43016-022-00559-y
-
Remote Sensing of Agriculture and Land Cover/Land Use Changes in South and Southeast Asian Countries.
*Vadrevu, K. P., Toan, T.L., Ray, S. S., & Justice, C. (Eds.). (2022). Remote Sensing of Agriculture and Land Cover/Land Use Changes in South and Southeast Asian Countries. Springer. https://doi.org/10.1007/978-3-030-92365-5
-
Using soil library hyperspectral reflectance and machine learning to predict soil organic carbon: Assessing potential of airborne and spaceborne optical soil sensing.
Wang, S., Guan, K., Zhang, C., Lee, D., Margenot, A. J., Ge, Y., Peng, J., Zhou, W., Zhou, Q., Huang, Y. (2022). Using soil library hyperspectral reflectance and machine learning to predict soil organic carbon: Assessing potential of airborne and spaceborne optical soil sensing. Remote Sensing of the Environment. https://doi.org/10.1016/j.rse.2022.112914
-
Unlocking large-scale crop field delineation in smallholder farming systems with transfer learning and weak supervision.
*Wang, S., Waldner, F., & Lobell, D. B. Unlocking large-scale crop field delineation in smallholder farming systems with transfer learning and weak supervision. (2022). arXiv preprint arXiv:2201.04771. https://arxiv.org/abs/2201.04771v1
-
Evaluating maize yield response to fertilizer and soil in Mexico using ground and satellite approaches.
*Campolo, J., Ortiz-Monasterio, I., Guerena, D., Lobell, D. Evaluating maize yield response to fertilizer and soil in Mexico using ground and satellite approaches. (2022). Field Crops Research. 276. https://doi.org/10.1016/j.fcr.2021.108393
-
Geo-CropSim: A Geo-spatial crop simulation modeling framework for regional scale crop yield and water use assessment.
*Bandaru, V., Yaramasu, R., Jones, C., Izaurralde, R., Reddy, A., Sedano, F., Daughtry, C., Becker-Reshef, I., Justice, C. Geo-CropSim: A Geo-spatial crop simulation modeling framework for regional scale crop yield and water use assessment. (2021). ISPRS Journal of Photogrammetry and Remote Sensing. 183 (34-53).
-
A model of asynchronous bi-hemispheric production in global agricultural commodity markets.
*Glauber, J., Miranda, M. A model of asynchronous bi-hemispheric production in global agricultural commodity markets. (2021). American Journal of Agricultural Economics. https://doi.org/10.1111/ajae.12241
-
Within-Field Rice Yield Estimation Based on Sentinel-2 Satellite Data.
Franch, B., Bautista, A.S., Fita, D., Rubio, C., Tarrazó-Serrano, D., Sánchez, A., Skakun, S., Vermote, E., Becker-Reshef, I., Uris, A. Within-Field Rice Yield Estimation Based on Sentinel-2 Satellite Data. (2021). Remote Sens. 13, 4095. https://doi.org/10.3390/rs13204095
-
The ARYA crop yield forecasting algorithm: Application to the main wheat exporting countries.
*Franch, B., Vermote, E., Skakun, S., Santamaria-Artigas, A., Kalecinski, N., Roger, J.C., Becker-Reshef, I., Barker, B., Justice, C., Sobrino, The ARYA crop yield forecasting algorithm: Application to the main wheat exporting countries. (2021). International Journal of Applied Earth Observation and Geoinformation. 104. https://doi.org/10.1016/j.jag.2021.102552.
-
Soil moisture retrieval improvement over agricultural fields by adding entropyalpha dual-polarimetric decomposition features.
*Akhavan, Z., Hasanlou, M., Hosseini, M., Becker-Reshef, I. (2021). Soil moisture retrieval improvement over agricultural fields by adding entropyalpha dual-polarimetric decomposition features. J. Appl. Remote Sens. 15(3), 034516. 10.1117/1.JRS.15.034516.
-
Daily Precipitation Frequency Distributions Impacts on Land-Surface Simulations of CONUS.
*Sarmiento, D., et al. Daily Precipitation Frequency Distributions Impacts on Land-Surface Simulations of CONUS. (2021) Frontiers in Water. 3. 10.3389/frwa.2021.640736.
-
Temporal integration of remote-sensing land cover maps to identify crop rotation patterns in a semiarid region of Argentina.
*Aoki, A., Robledo, J., Izaurralde, C., Balzarini, M. (2021). Temporal integration of remote-sensing land cover maps to identify crop rotation patterns in a semiarid region of Argentina. Agronomy Journal. DOI: 10.1002/agj2.20758
-
Soil Moisture Change Monitoring from C and L-band SAR Interferometric Phase Observations.
*Ranjbar, S., Akhoondzadeh, M., Brisco, B., Amani, M., Hosseini, M. (2021). Soil Moisture Change Monitoring from C and L-band SAR Interferometric Phase Observations. IEEE Journal of Selected Topics in Applied Earth Observations and Remote Sensing. DOI 10.1109/JSTARS.2021.3096063
-
A review of satellite-based global agricultural monitoring systems available for Africa.
*Nakalembe, C., Becker-Reshef, I., Bonifacio, R., Hua, G., Humber, M., Justice, C. Keniston, J., Mwangi, K., Rembold, F., Shukla, S., Urbano, F., Whitcraft, A., Li, F., Zappacosta, M., Jarvis, I., Sanchez, A. (2021). A review of satellite-based global agricultural monitoring systems available for Africa. Science Direct. 29. https://doi.org/10.1016/j.gfs.2021.100543
-
Land-use conversions from managed grasslands to croplands in Uruguay increase medium-term net carbon emissions to the atmosphere.
*Castaño-Sánchez, J., Izaurralde, R., Prince, S. (2021). Land-use conversions from managed grasslands to croplands in Uruguay increase medium-term net carbon emissions to the atmosphere. Journal of Land Use Science. 16(3), 240-259. 10.1080/1747423X.2021.1933227
-
Massive soybean expansion in South America since 2000 and implications for conservation.
*Song, XP., Hansen, M.C., Potapov, P. et al. Massive soybean expansion in South America since 2000 and implications for conservation. Nat Sustain (2021). https://doi.org/10.1038/s41893-021-00729-z
-
Two shifts for crop mapping: Leveraging aggregate crop statistics to improve satellite-based maps in new regions.
Kluger, D., Wang, S., Lobell, D. (2021). Two shifts for crop mapping: Leveraging aggregate crop statistics to improve satellite-based maps in new regions. Remote Sensing of Environment. 262. https://doi.org/10.1016/j.rse.2021.112488
-
Environmental and political implications of underestimated cropland burning in Ukraine.
Hall, J., Zibstev, S., Giglio, L., Skakun, S., Myroniuk, V., Zhuravel, O., Goldammer, J., Kussul, N.. (2021). Environmental and political implications of underestimated cropland burning in Ukraine. Environmental Research Letters. https://doi.org/10.1088/1748-9326/abfc04
-
A Comparison between Support Vector Machine and Water Cloud Model for Estimating Crop Leaf Area Index.
*Hosseini, M., McNairn, H., Mitchell, S., Robertson, L. D., Davidson, A., Ahmadian, N., Bhattacharya, A., et al. (2021). A Comparison between Support Vector Machine and Water Cloud Model for Estimating Crop Leaf Area Index. Remote Sensing, 13(7), 1348. MDPI AG. Retrieved from http://dx.doi.org/10.3390/rs13071348
-
An operational automated mapping algorithm for in-season estimation of wheat area for Punjab, Pakistan.
Khan, A., Hansen, M., Potapov, P., Adusei, B., Stehman, S., Steininger, M. (2021) An operational automated mapping algorithm for in-season estimation of wheat area for Punjab, Pakistan. International Journal of Remote Sensing. 42(10), 3833-3849. 10.1080/01431161.2021.1883200
-
Vegetation Monitoring Optimization With Normalized Difference Vegetation Index and Evapotranspiration Using Remote Sensing Measurements and Land Surface Models Over East Africa.
*Pervez, S., McNally, A., Arsenault, K., Budde, M., Rowland, J. (2020). Vegetation Monitoring Optimization With Normalized Difference Vegetation Index and Evapotranspiration Using Remote Sensing Measurements and Land Surface Models Over East Africa. https://doi.org/10.3389/fclim.2021.589981
-
An evaluation of Landsat, Sentinel-2, Sentinel-1 and MODIS data for crop type mapping. Science of Remote Sensing.
*Song, X., Huang, W., Hansen, M., Potapov, P. (2020). An evaluation of Landsat, Sentinel-2, Sentinel-1 and MODIS data for crop type mapping. Science of Remote Sensing. 3. https://doi.org/10.1016/j.srs.2021.100018
-
A slow rainy season onset is a reliable harbinger of drought in most food insecure regions in Sub-Saharan Africa.
*Shukla, S., Husak, G., Turner, W., Davenport, F., Funk, C., Harrison, L., Krell, N. (2020). A slow rainy season onset is a reliable harbinger of drought in most food insecure regions in Sub-Saharan Africa. PLOS ONE. https://doi.org/10.1371/journal.pone.0242883
-
Decomposition-Based Soil Moisture Estimation Using UAVSAR Fully Polarimetric Images.
Akhavan, Z., Hasanlou, M., Hosseini, M., McNairn, H. (2021). Decomposition-Based Soil Moisture Estimation Using UAVSAR Fully Polarimetric Images. Agronomy. 11(1), 145. https://doi.org/10.3390/agronomy11010145
-
Evaluating the Impact of the 2020 Iowa Derecho on Corn and Soybean Fields Using Synthetic Aperture Radar.
*Hosseini, M., Kerner, H., Sahajpal, R., Puricelli, E., Lu, Y., Lawal, A., Humber, M., Mitkish, M., Meyer, S., Becker-Reshef, I. (2020). Evaluating the Impact of the 2020 Iowa Derecho on Corn and Soybean Fields Using Synthetic Aperture Radar. Remote Sensing. 12(23), 3878. https://doi.org/10.3390/rs12233878
-
C-band synthetic aperture radar (SAR) imagery for the classification of diverse cropping systems.
Dingle Robertson, L., Davidson, A., McNairn, H., Hosseini, M., Mitchell, S., Abelleyra, D., Verón, S., et al. (2020). C-band synthetic aperture radar (SAR) imagery for the classification of diverse cropping systems. International Journal of Remote Sensing. https://doi.org/10.1080/01431161.2020.1805136
-
Changes in the drought sensitivity of US maize yields.
*Lobell, D., Deines, J.,Tommaso, S. (2020). Changes in the drought sensitivity of US maize yields. Nature Food. https://doi.org/10.1038/s43016-020-00165-w
-
High-Resolution Soybean Yield Mapping Across the US Midwest Using Subfield Harvester Data.
*Dado, W., Deines, J., Patel, R., Liang, S., Lobell, D. (2020). High-Resolution Soybean Yield Mapping Across the US Midwest Using Subfield Harvester Data. Journal of Remote Sensing. 12(21), 3471. https://doi.org/10.3390/rs12213471
-
Urgent and critical need for Sub-Saharan African countries to invest in Earth observation-based agricultural early warning and monitoring systems.
*Nakalembe, C. (2020). Urgent and critical need for Sub-Saharan African countries to invest in Earth observation-based agricultural early warning and monitoring systems. Environmental Research Letters. https://doi.org/10.1088/1748-9326/abc0bb
-
Assessing the Impact of ENSO on Agriculture Over Africa Using Earth Observation Data.
Sazib, N., Mladenova, I., Bolton, J. (2020). Assessing the Impact of ENSO on Agriculture Over Africa Using Earth Observation Data. Frontiers in Sustainable Food Systems. https://doi.org/10.3389/fsufs.2020.509914
-
Mapping Conservation Management Practices and Outcomes in the Corn Belt Using the Operational Tillage Information System (OpTIS) and the Denitrification–Decomposition (DNDC) Model.
Hagen, S., et al. (2020). Mapping Conservation Management Practices and Outcomes in the Corn Belt Using the Operational Tillage Information System (OpTIS) and the Denitrification–Decomposition (DNDC) Model. MDPI, Multidisciplinary Digital Publishing Institute Land Special Issue on Cropland Carbon. 9(11), 408. https://doi.org/10.3390/land9110408
-
Remote Sensing of Coconut Trees in Tonga Using Very High Spatial Resolution WorldView-3 Data.
Vermote, E.F., Skakun, S., Becker-Reshef, I., Saito, K. (2020). Remote Sensing of Coconut Trees in Tonga Using Very High Spatial Resolution WorldView-3 Data. Remote Sensing Journal. 12 (19), 3113. https://doi.org/10.3390/rs12193113
-
Mapping twenty years of corn and soybean across the US Midwest using the Landsat archive.
*Wang, S., Tommaso, S., Deines, J., Lobell, D. (2020). Mapping twenty years of corn and soybean across the US Midwest using the Landsat archive. (307). https://doi.org/10.1038/s41597-020-00646-4
-
Pre-season crop type mapping using deep neural networks. Computers and Electronics in Agriculture.
*Bandaru, V., Yaramasua, R., PNVR, K. (2020). Pre-season crop type mapping using deep neural networks. Computers and Electronics in Agriculture. (176). https://doi.org/10.1016/j.compag.2020.105664
-
PhenoCrop: An integrated satellite-based framework to estimate physiological growth stages of corn and soybeans.
*Bandaru, V., Yaramasua, R., PNVR, K., He, J., Sedano, F., Sahajpal, R., Wardlow, B.D., Suyker, A., Justice, C. (2020). PhenoCrop: An integrated satellite-based framework to estimate physiological growth stages of corn and soybeans. International Journal of Applied Earth Observation and Geoinformation. (92). https://doi.org/10.1016/j.jag.2020.102188
-
Integration of synthetic aperture radar and optical satellite data for corn biomass estimation.
Hosseini, M., McNairn, H., Mitchell, S., Dingle Robertson, L., Davidson, A., Homayouni, S. (2020). Integration of synthetic aperture radar and optical satellite data for corn biomass estimation. 7 (100857). https://doi.org/10.1016/j.mex.2020.100857
-
Algorithm and Data Improvements for Version 2.1 of the Climate Hazards Center’s InfraRed Precipitation with Stations Data Set.
*Funk, C., Peterson, P., Landsfeld, M., Davenport, F., Becker, A., Schneider U., Pedreros, D., McNally, A., Arsenault, K., Harrison, L., Shraddhanand, S. (2020). Algorithm and Data Improvements for Version 2.1 of the Climate Hazards Center’s InfraRed Precipitation with Stations Data Set. 67 (409-427). https://doi.org/10.1007/978-3-030-24568-9_23
-
A pan-African high-resolution drought index dataset. Earth System Science Data. 12 (753–769).
*Peng, J., Dadson, S., Hirpa, F., Dyer, E., Lees, T., Miralles, D., Vicente-Serrano, S., Funk, C. (2020). A pan-African high-resolution drought index dataset. Earth System Science Data. 12 (753–769). https://doi.org/10.5194/essd-12-753-202
-
Estimating and understanding crop yields with explainable deep learning in the Indian Wheat Belt.
Wolanin, A., Mateo-García, G., Camps-Valls, G., Gómez-Chova, L., Meroni, M., Duveiller, G., Liangzhi, Y., Guanter, L. (2020). Estimating and understanding crop yields with explainable deep learning in the Indian Wheat Belt. Environmental Research Letters 15 (2). https://doi.org/10.1088/1748-9326/ab68ac
-
Deriving high-spatiotemporal-resolution leaf area index for agroecosystems in the U.S. Corn Belt using Planet Labs CubeSat and STAIR fusion data.
*Kimm, H., Guan, K., Jiang, C., Peng, B., Gentry, L., Wilkin, S., Wang, S., Cai, Y., Bernacchi, C., Peng, J., Luo, Y. (2020). Deriving high-spatiotemporal-resolution leaf area index for agroecosystems in the U.S. Corn Belt using Planet Labs CubeSat and STAIR fusion data. Remote Sensing of Environment. 239. https://doi.org/10.1016/j.rse.2019.111615
-
Detecting In-Season Crop Nitrogen Stress of Corn for Field Trials Using UAV- and CubeSat-Based Multispectral Sensing.
*Cai, Y., Guan, K., Nafziger, E., Chowdhary, G., Peng, B., Jin, Z., Wang, S., Wang, S. (2020). Detecting In-Season Crop Nitrogen Stress of Corn for Field Trials Using UAV- and CubeSat-Based Multispectral Sensing. IEEE Xplore Digital Library. 12 (12). https://ieeexplore.ieee.org/document/8950295
-
Satellites reveal a small positive yield effect from conservation tillage across the US Corn Belt.
*Deines, J., Lobell, D., Want, S. (2020). Satellites reveal a small positive yield effect from conservation tillage across the US Corn Belt. Environmental Research Letters. 14 (12). https://iopscience.iop.org/article/10.1088/1748-9326/ab503b
-
Quantifying carbon for agricultural soil management: from the current status toward a global soil information system.
*Baldock, J., Burgess, R., Collier, S., Creque, J., DeLonge, M., Dungait, J., Ellert, B., Frank, S., Goddard, T., Govaerts, B., Grundy, M., Henning, M., Izaurralde, R.C., Jahn, M., Madaras, M., McConkey, Mulhern, W., Paustian, K., B., Porzig, E., Rice, C., Searle, R., Seavy, N., Skalsky, R. (2019). Quantifying carbon for agricultural soil management: from the current status toward a global soil information system. Carbon Management. 10:6 (567-587). https://doi.org/10.1080/17583004.2019.1633231
-
The GEOGLAM crop monitor for AMIS: Assessing crop conditions in the context of global markets.
*Becker-Reshef, I., Barker, B., Humber, M., Puricelli, E., Sanchez, A., Sahajpal, R., McGaughey, K., Justice, C., Baruth, B., Wu, B., Prakash, A., Abdolreza, A., Jarvis, I. 2019. The GEOGLAM crop monitor for AMIS: Assessing crop conditions in the context of global markets. Global Food Security. 23, 173-181. https://doi.org/10.1016/j.gfs.2019.04.010
-
A multi-temporal binary-tree classification using polarimetric RADARSAT-2 imagery.
Huang, X., Liao, C., Xing, M., Ziniti, B., Wang, J., Shang, J., … Torbick, N. (2019, October 30). A multi-temporal binary-tree classification using polarimetric RADARSAT-2 imagery. Remote Sesning of Environment, 235. https://doi.org/10.1016/j.rse.2019.111478
-
No Pixel Left behind: Toward Integrating Earth Observations for Agriculture into the United Nations Sustainable Development Goals Framework.
*Whitcraft, Alyssa K., et al. “No Pixel Left behind: Toward Integrating Earth Observations for Agriculture into the United Nations Sustainable Development Goals Framework.” Remote Sensing of Environment, Elsevier, 31 Oct. 2019. https://doi.org/10.1016/j.rse.2019.111470
-
Acute Water-Scarcity Monitoring for Africa.
*McNally, A.; Verdin, K.; Harrison, L.; Getirana, A.; Jacob, J.; Shukla, S.; Arsenault, K.; Peters-Lidard, C.; Verdin, J.P. 2019. Acute Water-Scarcity Monitoring for Africa. Water 11, 1968. https://www.mdpi.com/2073-4441/11/10/1968
-
Integrating satellite and climate data to predict wheat yield in Australia using machine learning approaches.
Cai, Y., Guan, K., Lobell, D., Potgieter, A., Wang, S., Peng, J., ... Peng, B. (2019). Integrating satellite and climate data to predict wheat yield in Australia using machine learning approaches. Elsevier Agricultural and Forest Meteorology, 274, 144-159. https://doi.org/10.1016/j.agrformet.2019.03.010
-
Winter Wheat Yield Assessment from Landsat 8 and Sentinel-2 Data: Incorporating Surface Reflectance, Through Phenological Fitting, into Regression Yield Models.
*Skakun, S.; Vermote, E.; Franch, B.; Roger, J.-C.; Kussul, N.; Ju, J.; Masek, J. 2019. Winter Wheat Yield Assessment from Landsat 8 and Sentinel-2 Data: Incorporating Surface Reflectance, Through Phenological Fitting, into Regression Yield Models. Remote Sens. 11, 1768. https://doi.org/10.3390/rs11151768
-
Transitioning from MODIS to VIIRS: an analysis of inter-consistency of NDVI data sets for agricultural monitoring.
Skakun S., Justice C., Vermote E., & Roger J.-C. (2018). Transitioning from MODIS to VIIRS: an analysis of inter-consistency of NDVI data sets for agricultural monitoring. International Journal of Remote Sensing (2016 IF:1.724; 5-year IF: 1.986), vol. 39, no. 4, pp. 971-992. doi: 10.1080/01431161.2017.1395970
-
Fusion of Moderate Resolution Earth Observations for Operational Crop Type Mapping.
*Torbick, N., Huang, X., Ziniti, B., Johnson, D., Masek, J. and Reba, M. 2018. Fusion of Moderate Resolution Earth Observations for Operational Crop Type Mapping. Remote Sens. 10(7), 1058. https://doi.org/10.3390/rs10071058
-
Recognizing the famine early warning systems network: over 30 years of drought early warning science advances and partnerships promoting global food security.
Funk, C., Shukla, S., Thiaw, W.M., Rowland, J., Hoell, A., McNally, A., … Verdin, J. (2019). Recognizing the famine early warning systems network: over 30 years of drought early warning science advances and partnerships promoting global food security. American Meteorological Society. https://journals.ametsoc.org/doi/10.1175/BAMS-D-17-0233.1
-
Assessing Conflict Driven Food Security in Rakhine, Myanmar with Multisource Imagery.
Huang, X., Ziniti, B., and Torbick, N. 2019. "Assessing Conflict Driven Food Security in Rakhine, Myanmar with Multisource Imagery," Land, MDPI, Open Access Journal, 8(6), 1-11. https://doi.org/10.3390/land8060095
-
Remote sensing based yield monitoring: Application to winter wheat in United States and Ukraine.
*Franch, B., Vermote, E. F., Skakun, S., Roger, J. C., Becker-Reshef, I., Murphy, E., & Justice, C. 2019. Remote sensing based yield monitoring: Application to winter wheat in United States and Ukraine. International Journal of Applied Earth Observation and Geoinformation, 76, 112-127. https://doi.org/10.1016/j.jag.2018.11.012
-
Impact of the Revisit of Thermal Infrared Remote Sensing Observations on Evapotranspiration Uncertainty— A Sensitivity Study Using AmeriFlux Data.
Guillevic, P.C., Olioso, A., Hook, S.J., Fisher, J.B., Lagouarde, J.P. and Vermote, E.F. 2019. Impact of the Revisit of Thermal Infrared Remote Sensing Observations on Evapotranspiration Uncertainty—A Sensitivity Study Using AmeriFlux Data. Remote Sensing, 11(5). https://doi.org/10.3390/rs11050573
-
Hydrologic and Agricultural Earth Observations and Modeling for the Water-Food Nexus.
*McNally, A., McCartney, S., Ruane, A., Mladenova, I., Whitcraft, A., Becker-Reshef, I., Bolten, J.D., Peters-Lidard, C., Rosenzweig, C. and Schollaert Uz, S. 2019. Hydrologic and Agricultural Earth Observations and Modeling for the Water-Food Nexus. Front. Environ. Sci. https://doi.org/10.3389/fenvs.2019.00023
-
Nutrition sensitive value chains: theory, progress, and open questions.
Allen, S., de Brauw, A. (2018). Nutrition sensitive value chains: theory, progress, and open questions. Glob. Food Secur. 16, 22–28. doi: 10.1016/j.gfs.2017.07.
-
Characterizing agricultural drought in the Karamoja subregion of Uganda with meteorological and satellite-based indices.
Nakalembe, C. 2018. Characterizing agricultural drought in the Karamoja subregion of Uganda with meteorological and satellite-based indices. Natural Hazards, 91(3), 837-862. https://doi.org/10.1007/s11069-017-3106-x
-
Validation of the LaSRC cloud detection algorithm for Landsat 8 images.
Skakun, S., Vermote, E.F., Roger, J.C., Justice, C.O. and Masek, J.G. 2019. Validation of the LaSRC cloud detection algorithm for Landsat 8 images. IEEE Journal of Selected Topics in Applied Earth Observations and Remote Sensing 12(7), 2439 - 2446. https://ieeexplore.ieee.org/document/8638529
-
Strengthening agricultural decisions in countries at risk of food insecurity: The GEOGLAM Crop Monitor for Early Warning.
*Becker-Reshef, I., Justice, C., Barker, B., Humber, M., Rembold F., Bonifacio, R., Zappacosta, M., Budde, M., Magadzire, T., Shitote, C., Pound, J., Constantino, A., Nakalembe, C., Mwangi, K., Sobue, S., Newby, T., Whitcraft, A., Jarvis, I., Verdin, J. 2020. Strengthening agricultural decisions in countries at risk of food insecurity: The GEOGLAM Crop Monitor for Early Warning. Remote Sensing of the Environment, 237, 111556, https://doi.org/10.1016/j.rse.2019.111553
-
A comparison of global agricultural monitoring systems and current gaps.
*Fritz, S., See, L., Laso Bayas, J.C., Waldner, F., Jacques, D., Becker-Reshef, I., Whitcraft, A., Baruth, B., Bonifacio, R., Crutchfield, J., Rembold, F., Rojas, O., Schucknecht, A., Van der Velde, M., Verdin, J., Wu, B., Yan, N., You, L., Gilliams, S., Mücher, S., Tetrault, R., Moorthy, I., McCallum, I. 2019. A comparison of global agricultural monitoring systems and current gaps. Agricultural Systems, 168, 258-272. https://doi.org/10.1016/j.agsy.2018.05.010
-
Information theoretic evaluation of satellite soil moisture retrievals.
Kumar, S. V., Dirmeyer, P. A., Peters-Lidard, C. D., Bindlish, R., & Bolten, J. (2018). Information theoretic evaluation of satellite soil moisture retrievals. Remote Sensing of Environment, 204, 392-400. https://doi.org/10.1016/j.rse.2017.10.016